Introducing Visual Browsing
What, you may ask, is visual browsing? Loosely defined, visual browsing user interfaces are those that let people navigate visual content—that is, search for content using pictures. We will discuss four types of visual browsing:
- browsing images or photos using text attributes or tags—for example, on Google Images and Flickr
- using images in queries to describe attributes that are hard to describe with text—as on Like.com and Zappos
- using images to facilitate wayfinding and navigation in the real world—such as on Google Maps and Photosynth
- collecting and browsing images on mobile devices—like on RedLaser and NeoReader
Each of these approaches to visual browsing supports different goals for searchers and introduces its own unique challenges, design directions, and best practices, which we’ll explore next.
Browsing Images Using Text Attributes or Tags
While there are many image-centric sites, the majority of the Web’s image content is on either Google Images or Flickr. No discussion of visual browsing would be complete without mentioning these two sites. When it comes to finding images that are published somewhere on the Web, Google Images is the site most people go to. Google uses a proprietary algorithm to assign keywords describing each image, then index and rank all of the images in their enormous database. They also capture a thumbnail of each image and store it along with the source URL.
While Google Images is undeniably a very useful site, it exemplifies some of the challenges of automated image finding. The primary mechanism for finding images is a text-only query, which perfectly demonstrates the impedance mismatch that exists between text and image content. Because no single keyword can adequately describe all of the pixels that make up an image—and, most of the time, the keywords that surround an image on a page provide an incomplete description at best—Google Images interprets search queries very loosely. Typically, it interprets multiple keywords using an OR instead of the usual and expected AND operator. As a consequence, it omits some keywords from the query without letting the searcher know explicitly what is happening.
On the one hand, because Google Images interprets search queries in a such a loose fashion, it rarely returns zero search results. On the other hand, image searches sometimes give us strange and unpredictable results, while making it challenging to understand what went wrong with our queries and how to adjust them to find the images we’re really looking for. Figure 1 shows an example—the search results for Africa Thailand elbow Indian.
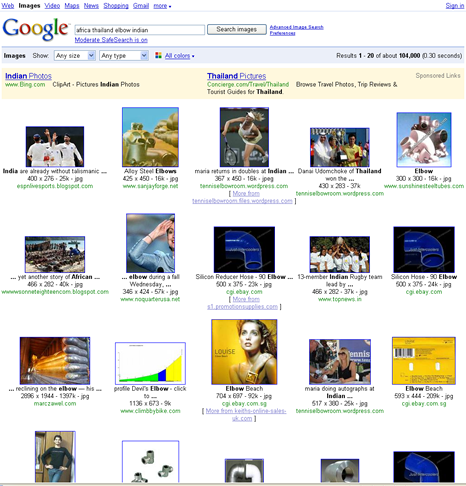
Judging from the search results, Google Images seems to have omitted keywords randomly from the query, without indicating to the searcher it has done so. Of course, one could argue that getting such poor results for a query is an example of garbage in/garbage out. However, we think this example demonstrates that the keywords Google takes from the surrounding content on a Web page—and possibly some Web pages that link to it—do not always match an image. While an image may technically be located at the intersection of Africa, Thailand, elbow, and Indian, this by no means guarantees that the image would actually show Indian warriors demonstrating the perfect Mai Thai elbow-strike technique on the plains of the Serengeti.
Plus, a computer-generated index usually makes no distinction between the different possible meanings of an image’s surrounding keywords—for example, a search engine can’t tell when an image appears in juxtaposition to text to add humor. Nor do automated indexing engines handle images well when they have purposes other than providing content—such as images that add visual appeal or bling or those for marketing or advertising. Such image content is, at best, orthogonal to its surrounding text.
Image search filters that are based on text are, at the moment, not particularly robust. For example, Figure 2 shows Google Images search results for iPhone with some filters applied. As you can see, the resulting images have little to do with the iPhone.
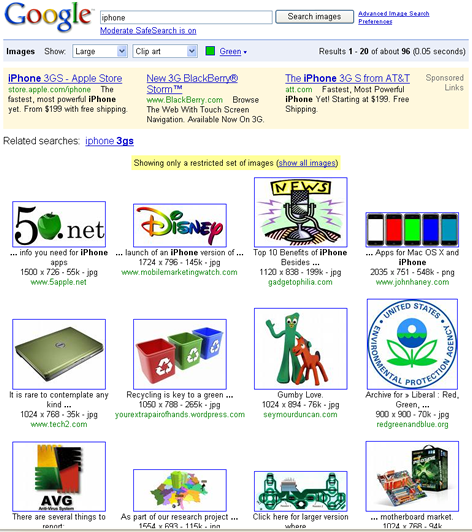
As a photo-hosting site, Flickr enjoys the distinct advantage of having people rather than computers look at, interpret, and tag the images they create and view. Users can search for images by their tags. While Flickr is not a perfect image-searching system, it provides an entirely subjective, human-driven method of interpreting and describing images. Why is that valuable?
According to Jung’s conception of the collective unconscious, we can assume all individuals experience the same universal, archetypal patterns. These archetypes influence our innate psychic predispositions and aptitudes, as well as our basic patterns of human behavior and responses to life experience. Likewise, at the root of all folksonomies is the inherent assumption that people—when we look at them collectively—tend to respond in similar ways when presented with the same stimuli. Simply put, people looking for images of cats would be quite happy to find the images that a multitude of other people have taken the time to tag with the word cat.
As Gene Smith describes in his book, Tagging: People-Powered Metadata for the Social Web, folksonomies and tagging usually work quite well—in most cases, nicely resolving the kinds of issues that occur in Google Images due to auto-indexing, which we described earlier. Unfortunately, the human element also introduces its own quirks along the way. Take a look at the example of a Flickr image shown in Figure 3.
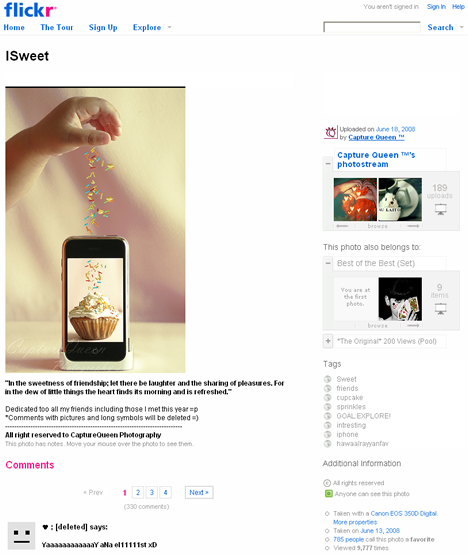
Among almost 2 million Flickr images tagged iPhone, Flickr considers this image one of the most interesting, with 785 favorites and 37 comments. As you can see at the bottom of the page, one of the problems with having a community-driven popularity algorithm is that some people post nonsense comments just to be able to say they’ve added a comment to a popular item.
Another problem has to do with tags that are strictly personal. For example, tags like GOAL:EXPLORE! and hawaalrayyanfav have meaning only to the person who actually tagged the item—and little or nothing to the population at large. On the other hand, adding tags like funny and humor is actually quite useful, because computers don’t recognize humor at all. In fact, humor is the aspect of image description where algorithm-based search results fail most spectacularly. We’ll talk more about folksonomies, controlled vocabularies, and searching for tags in future columns. For now, we’ll just say that neither way of finding images—through text tags or associated surrounding text—is perfect, and there is a great deal of room for improvement, perhaps through combining both search approaches, using a single algorithm and a user interface that does not yet exist.